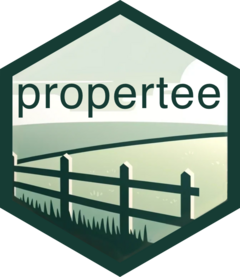
Design based sandwich SEs for differences of Hajek estimators
Source:vignettes/design_based_SEs.Rmd
design_based_SEs.Rmd
We’ve promised to provide sandwich-type SEs for estimates of treatment effect, with stacking of estimated equations as appropriate to address possible use of separate regression fits to estimate covariance adjustment terms and then to estimate marginal treatment effects. Often ``sandwich standard error’’ conjures an expectation of standard errors that are model-based in the sense of assuming i.i.d. data and making inference conditional on the values of treatment assignments , importance or frequency weights , and covariates , but not the values of potential responses . (This document takes to range over intact clusters.) With the i.i.d assumption, these model-based SEs are valid even if the design specification isn’t, provided that the outcome model is well-specified. The role of the study design is to provide analysis weights which, if respected, ensure desired interpretations of marginal effect estimators if the -model happens to be misspecified.
An alternative would be to offer sandwich standard errors under a design-based interpretation, differing from the above principally in terms of conditioning on
where is a given partition of {1,, n} encoding a stratification. Of particular interest are standard errors for Hajek estimators, i.e. estimators of form and their differences The case weights are the products of a frequency or importance weight and, as appopriate, a weighting factor representing a reciprocal of ’s probability (or odds) of assignment to (or to one of {}); in general differ by . With for , is the natural estimate of the effect of treatment within the experimental or quasiexperimental study sample, . This is also known as the sample average treatment effect. If the experimental observations are samples from a broader super-population, with sample inclusion probabilities , then setting for makes into the corresponding natural estimate of the population average treatment effect.
In one special case a design-based SE for has been available for some time, with an optional interpretation in terms of M-estimation emerging more recently. Suppose:In this case the variance estimate used in the 2 sample test without pooling of variances validly estimates the variance of , in the following sense. If is observed only when while is observed only when , then is not identified; as far as the data are concerned, it could lie anywhere between , where . Assuming , then the ordinary unpooled variance is unbiased for the design-based variance (conditional variance given ) of . If on the other hand , then the expected value of this ordinary unpooled variance exceeds ’s design-based variance . The possibilities and being exhaustive, by Cauchy-Schwartz, the expected value of the unpooled variance can be no less than ; it is valid as a possibly conservative estimate in that it cannot be negatively biased.
As shown by , in this case the same variance estimate coincides with the HC2 flavor of the Huber-White estimate. As a result, it’s sometimes said that ordinary sandwich or cluster-robust variance estimates admit of design-based interpretations provided that one uses the HC2 form. I suspect this works only in special cases, however, perhaps not going far beyond the one delimited above.
The use of the Neyman insight to get design-based SEs through Huber-White covariance estimates intended for model-based interpretation has several important limitations.
The M-estimation framework promises solutions to many or all of these impediments.The Hajek estimators , , admit of being defined as the unique multivariate root of the estimating function
The information matrices corresponding to are diagonal. For a sandwich estimate of variance, then, we only need estimates of the covariance of~. In the one-stratum case with multiple representatives of conditions and , we can simply apply Neyman’s two-sample unpooled variances SE to observations and . Impediment~ is solved.
For standard errors of the difference of Hajek estimators within a subgroup , we can simply fold the subgroup indicator into the weights, weighting by as opposed to . This solves the first part of Impediment~.
(The remaining piece of Impediment~ is the need for estimates of where and are observed only when , all and . I haven’t had the opportunity to sit down and try to work this out, but I’m optimistic that this can be handled with straightforward extensions of the Neyman insight.)
Under the design-based interpretation, variances and covariances of quantile regression estimating functions — for a quantile regression with covariates and observations per cluster , — are likely to be estimable in much the same way as variances and covariances of estimating functions for other forms of regression. In any case, there will be no need for estimation of a sparsity parameter. The impediment is removed.
(Quantile regression doesn’t bear any of its usual interpretations under conditioning on , but that doesn’t matter for purposes of using it to estimate slopes in an RDD.)
Now consider Impediment~, strata in which one or both of the conditions being contrasted have only one representative, . Consider the situation without covariates.
As there is independence across if not within strata, the B matrices corresponding to~ are sums of stratum-wise contributions For any stratum and condition s.t. , is not estimable, just as the variance is not estimable. On the other hand, the second moment estimable, by it sample realization : in M-estimation, we’re entitled to treat is a fixed constant, not a random variable. Since the second moment bounds the variance from above, is a safe, conservative variance estimate.
M-estimation doesn’t help us to estimate but these terms aren’t identified even for large strata with multiple representatives of either condition. When interest is in the difference , conservatism leads us to ``impute’’ the covariance value that maximizes the variance of that difference, that corresponding to a correlation of 1 between and . We can follow the same principle when approaching the problem from the perspective of -estimation, whatever the representation of conditions and 0 within stratum .
In separate hand-written notes I begin fleshing this out into methods of estimating design-based second moments of first differences of the estimating functions that define the Hajek estimators, which can in turn be used to approximate the corresponding B-matrix terms; extending this work might be a suitable problem for a PhD student.
%
If covariate are included in a linear regression specification with unit weights — that is, the specification that without would have engendered estimation of Hajek estimator differences as in — then B-matrix estimation can still be done just as it is without the covariates. The A matrices are no longer diagonal, but are otherwise straightforward. Combining estimates of these A and B matrices would give legitimate standard errors for differences of Hajek estimators as before, but now with adjustment for covariates.
%
Another approach to covariates is to have fit their coefficients in a separate and prior regression fit — perhaps a robust linear fit; or perhaps a binary regression fit — and then to contrast Hajek aggregates of residuals from these fits . This approach calls for stacked estimating equations, with accompanying complications to both the A and the B matrix. We might as well consider it in tandem with challenges and .
To draw a distinction between experimental versus non-experimental observations, let be a partition of a nonempty subset of , so that some but not necessarily all clusters 1,, fall into some stratum ; these clusters are then the experimental or quasi-experimental sample. Re-define to contain similar information as above but also full information on clusters falling outside of : One now needs A and B matrices for estimating functions of the Hajek estimator and the regression estimator simultaneously. Given that we’re ultimately interested only to estimate covariances for the Hajek differences, simplified expressions in terms of A- and B-submatrices are available . Further simplification of B matrices is possible given our conditioning on in ; the B matrix continues to be a sum of form~, with clusters making no contribution. (The design-based interpretation has no bearing on calculation of matrices.)