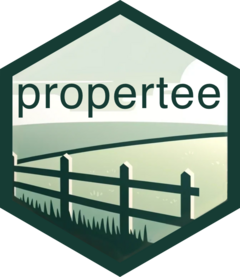
propertee vs. geex
Joshua Wasserman
2024-11-20
Source:vignettes/geex_vs_propertee.Rmd
geex_vs_propertee.Rmd
Overview
We provide basic validation for the model-based sandwich standard errors provided in by comparing them to estimates from the package (Saul and Hudgens, 2020). In two examples, one with and one without clustering, we estimate the standard error of an intent-to-treat (ITT) effect estimate that has been adjusted for covariates using a prior covariance model. In the M-estimation framework, the prior covariance model defines one set of estimating equations, and the covariate-adjusted intent-to-treat effect estimate defines another. One can stack them togther and use the package to obtain joint estimates of the model coefficients and ITT effect, or one can fit the covariance model first, then use the package to feed necessary information about the first-stage estimating equations to variance estimation for the ITT effect estimate. We demonstrate the equivalence of these two appraoches below.
Example with non-clustered data
set.seed(980)
n <- 60
cmod_df <- data.frame(
"uid" = seq_len(n),
"z" = c(rep(0, 3 * n/4), rep(1, n/4)),
"x1" = rnorm(n, sd = 2),
"x2" = rep(c(rep(0, n/4), rep(1, n/4)), 2)
)
eps <- rnorm(nrow(cmod_df))
theta <- c(75, 0.1, -0.5, 2.5)
cmod_df$y <- model.matrix(~ x1 + x2 + z, cmod_df) %*% theta + eps
cmod_df$in_q <- c(rep(0, n/2), rep(1, n/2))
q_df <- cmod_df[cmod_df$in_q == 1,]
cmod <- lm(y ~ x1 + x2, cmod_df)
spec <- rct_spec(z ~ unitid(uid), q_df)
damod <- lmitt(y ~ 1, specification = spec, data = q_df, weights = ate(spec), offset = cov_adj(cmod))
estFun <- function(data){
function(theta) {
# covariance model eqns
Xstar <- model.matrix(y ~ x1 + x2, data)
cmod_eqns <- drop(data$y - Xstar %*% theta[1:3]) * Xstar
# itt model eqns
X <- model.matrix(y ~ x1 + x2, data)
Z <- model.matrix(y ~ z, data)
if (data$in_q == 1) {
damod_eqns <- drop(data$weight * (data$y - X %*% theta[1:3] - Z %*% theta[4:5])) * Z
} else {
damod_eqns <- rep(0, 2)
}
out <- c(cmod_eqns, damod_eqns)
return(out)
}
}
geexRes <- geex::m_estimate(estFun,
data = cbind(cmod_df,
"weight" = c(rep(1, n/2), ate(spec, data = q_df))),
root_control = geex::setup_root_control(start = rep(0.1,5)))
print(paste("geex estimate of var(tau_hat):", round(geexRes@vcov[5,5], 8)))
print(paste("propertee estimate of var(tau_hat):",
round(vcov_tee(damod, type = "CR0", cadjust = FALSE)[2,2], 8)))
Example with clustered data
set.seed(50)
nc <- 4
mi <- 20
cmod_df <- data.frame(
"cid" = rep(seq_len(4), each = mi),
"uid" = rep(seq_len(mi), nc),
"z" = c(rep(0, 3 * mi), rep(1, mi)),
"x1" = rbinom(nc * mi, 1, 0.5),
"x2" = rnorm(nc * mi)
)
theta <- c(75, 7.5, -1, 2.5)
error_sd <- round(runif(nc, 1, 3), 1)
icc <- 0.2
eps <- rnorm(nrow(cmod_df))
Sigma <- matrix(0, nrow = nrow(cmod_df), ncol = nrow(cmod_df))
for (i in (seq_len(nc) - 1)) {
msk <- cmod_df$cid == (i + 1)
Sigma[msk, msk] <- diag(error_sd[i + 1] - icc, nrow = sum(msk)) + icc
}
A <- chol(Sigma)
eps <- t(A) %*% eps
cmod_df$y <- model.matrix(~ x1 + x2 + z, cmod_df) %*% theta + eps
cmod_df$in_q <- c(rep(0, mi), rep(1, 3 * mi))
q_df <- cmod_df[cmod_df$in_q == 1,]
cmod <- lm(y ~ x1 + x2, cmod_df)
spec <- rct_spec(z ~ cluster(cid), q_df)
damod <- lmitt(y ~ 1, specification = spec, data = q_df, weights = ate(spec), offset = cov_adj(cmod))
clusterEstFunc <- function(data){
function(theta) {
# covariance model eqns
Xstar <- model.matrix(y ~ x1 + x2, data)
cmod_agg_func <- ifelse(dim(Xstar)[2] > 1, colSums, sum)
cmod_eqns <- cmod_agg_func(drop(data$y - Xstar %*% theta[1:3]) * Xstar)
# itt model eqns
X <- model.matrix(y ~ x1 + x2, data)
Z <- model.matrix(y ~ z, data)
damod_agg_func <- ifelse(dim(Z)[2] > 1, colSums, sum)
if (unique(data$in_q) == 1) {
damod_eqns <- damod_agg_func(
drop(data$weight * (data$y - X %*% theta[1:3] - Z %*% theta[4:5])) * Z)
} else {
damod_eqns <- rep(0, 2)
}
out <- c(cmod_eqns, damod_eqns)
return(out)
}
}
geexRes <- geex::m_estimate(clusterEstFunc,
data = cbind(cmod_df,
"weight" = c(rep(1, mi), ate(spec, data = q_df))),
units = "cid",
root_control = geex::setup_root_control(start = rep(0.1,5)))
print(paste("geex estimate of var(tau_hat):", round(geexRes@vcov[5,5], 8)))
print(paste("propertee estimate of var(tau_hat):",
round(vcov_tee(damod, type = "CR0", cadjust = FALSE)[2,2], 8)))